By devtodev lead analyst Vasiliy Sabirov
Analytics in most cases is simply the monitoring of key metrics: DAU, MAU, WAU, ARPU, ARPPU and other abbreviations. Basic analytics metrics represent the 20% of the functional of analytic systems, which provide 80% of the result.
But are these 80% enough?
If not, then our article is for you. We will talk about some of the metrics that are also worthwhile to keep in mind if you want to fully understand all the processes that occur in your application.
Acquisition Metrics
So, users start to use your application. You measure the number of new users (New Users), the total number of users on a particular date (Total Users). You calculate the price to attract users (CPI), the effectiveness of your investment (ROI).
But in order to start the flow of traffic from the partner, first you have to find a partner, sign the contract (agreement with lawyers is often not so fast to be done), integrate and agree on everything. That is to spend both time and money either to pay your employees or on one-time payment to the partner (this also happens). Therefore we recommend to calculate not only the usual CPI, but also the effective cost of user acquisition (eCPI), which includes all third-party costs.
Accordingly, it is better to calculate ROI by putting eCPI in the denominator. Thus, you get eROI. And it may well be so, that on the basis of eCPI and eROI you choose completely different partners.
Retention
Here the classic retention for 1 day, 7 days, 28 days, 60 days and so on is usually measured. Some prefer rolling retention, when the user is considered to be retained in 7 days, if he returned to the application on the seventh day or later.
Do you measure the retention of the first session? Do you examine the FTUE (First Time User Experience)? But the first session defines almost everything. You will be amazed when you see how many users leaving you already when passing the tutorial.
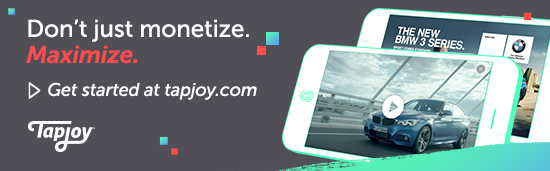
And since we’re talking about the tutorial, it is recommended to measure the tutorial retention. In particular, it is possible to use the Tutorial Steps Report.
For you each item in the menu - is just an item of the menu, and for a whole bunch of new users that come to you every day, it is the point of bifurcation, where users may make a final decision about leaving. That is why the tutorial can and should be divided into the smallest steps, you are to track those steps where users leave and optimize the bottlenecks. Your 1-day retention is necessary to be optimized from the very beginning - namely, from the optimization of the tutorial.
It is also recommended to use such a metric as 28/1-retention (28-days retention divided by 1-day retention). It is obtained from two metrics already familiar to you, but it will help you to understand how many users from those that remain active after 1 day, will remain active after 28 days. Might happen that the majority of users leave on the first day, and those who stay, stay with you for a long time (in this case 28/1-retention is higher). So, all you have to do is to optimize the retention of the first day.
It’s like the transition from conventional to Bayesian probability.
What kind of users can be called active? Usually referred to as active, those users who were in the application during the last week (sometimes two weeks). And how shall we call a user after that? Inactive? Wrong. Sleeping. If the user didn’t log in the application for a week, it does not mean that he is not coming back. Give him a chance and select sleeping users in a separate category: dormant users. Define the boundaries yourselves: for example, the absence of login within a period from one week to six months. Sleeping users can still be brought back and the less user is “asleep”, the higher the chance is. Push-notifications, deep-linking, interesting updates, regular assignments and tournaments are your first-aid helpers.
And if the user suddenly “wakes up” and returns to the application, such users may be called returning users, and now you certainly shouldn’t let them leave (daily quests, gifts for the return, customized tune of difficulty of the levels, and so on).
Engagement Metrics
Typically, those are the metrics of the size of your application: DAU, WAU, MAU, Users Online. Sometimes they also include the average length of session and Lifetime.
And if you divide the DAU by MAU, we will get an interesting metric called Sticky Factor.
It shows the regularity of the custom launches during the month: the more regular users launch the app, the higher the Sticky Factor is.
If we assume that every user launches the game every day for a month, then DAU = WAU = MAU, and hence, Sticky Factor = 100%. But is this achievable, and whether or not this figure affects the monetization?
To answer this question, we analyzed about 100 mobile games, and calculated the average Sticky Factor for the first three months of 2015.
The average value of the indicator equal to 18%, while the minimum value was 4%, and the maximum is 37%.
After that we calculated the correlation between the Sticky Factor indicator and the income. Correlation was 50-60% depending on the category of the game. This suggests that the relationship between the income and the Sticky Factor certainly exists, but it is not strong enough to be able to say that only the Sticky Factor affects the income.
Many people measure the average length of the session. But the number of sessions per active user per day (Sessions/DAU) is not measured by all of them. And yet it is also an excellent indicator of the regularity of logins. Trevor McCalmont cites the following reference values of this magnitude:
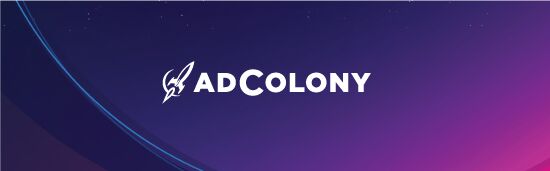
- good value - is 3 sessions per user per day
- for games with a longer session (RPG, for example) - 2 sessions
- for games with short gaming session (casual, runner) - 4-5 sessions.
We see that for games with short session, the value is higher, and for games with long gaming session - it is lower. Consequently, we may talk about another metric - playing time per user per day (Average Playing Time Per Day). It allows you to abstract from the average length of session and number of sessions and perfectly describes user’s interest in the game.
We also recommend you to measure the Frequency by Day: the frequency of user logins, depending on the time from their first visit. As a rule, this value decreases. In some cases, slowly but surely, in other cases rapidly from the first day. Proper use of this metric will allow you to predict the future of user’s leaving the game, and when finding such users, we are sure you will do everything to keep them in your project.
Monetization
Here total income is often measured (Gross, Revenue) and averages: ARPU, ARPPU, Average Check.
Also, the number and proportion of paying users is measured: Paying Users, Paying Share, Paying Conversion.
Do you know how many users, of those who made a payment today, made it for the first time? We recommend to use New Paying Users metric. The probability of the second payment after the first one equals to approximately 70% - 90%. And the probability of the third payment after the second one is even higher. The further, the higher the probability is. Consequently, the important thing to do is to get the user to make the first payment. And then it will be easier. And the metric described will help you to understand how many users convert into paying users over the period.
We have already talked about the importance of studying FTUE. For the purposes of monetization is just as important to also study FTPUE (First Time Paying User Experience). It is important to understand what motivates users to convert from non-paying to paying, what period of time does this conversion take, which events in the game get involved, what is the balance of game currency when it takes place. In particular, it is necessary to look at what people bought, when made their first payment - First-Selling Items. They may differ from the most popular items. Their function is in the fact that the user has made the first payment. After examining what exactly in these subjects were able to attract a user, you will be able to use this experience in various promotions and to take into account when making decisions on monetization.
Also curious to consider the conversion of the in-game store: the number of purchases divided by the number of store openings. It is easy to estimate, if you integrate the custom event for the store opening. And this metric reveals to you the way to optimize the store: you may want to arrange the items in a different order, change prices or simply rewrite the descriptions and redraw the icons.
In general, the economy of the game can be studied for a long time. There are many methods that work.
Social Metrics
If users like the game or app, they gladly share information about it, and you receive feedback in the form of free organic traffic.
It is possible to measure the number of invitations sent in average by one user: Invites Sent. It’s not so difficult to measure: you may either generate a unique reference and count them, or simply embed the appropriate custom event.
To measure the impact of social factors, there is the so-called K-factor. For web projects, it is usually calculated as Invites Sent, multiplied by the conversion from invitation to registration. For mobile projects it is usually difficult to estimate the number of users who saw the invitation (usually it is not generated as a unique reference but as a post at social network), so we offer the following formula:
K-factor = (New users from the Organic Channel) / (DAU - New users)
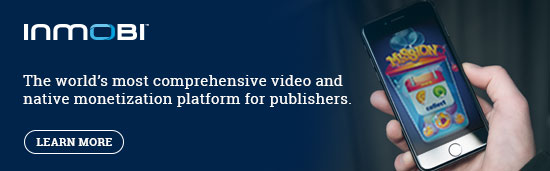
What tells us K-factor? In fact, it is the average number of friends invited by one active user. In particular, the Wooga company announced its K-factor, it equals to 0.92. This means that one active player invites an average of 0.92 of a friend. So for each 100 active players in the game comes 92 new free players.
This also means that Wooga can easily multiply LTV of their player by (1+0.92) and have a so-called Social LTV, which is almost twice as much. And Social LTV better characterizes the amount that brings one player: he not only pays himself, but also invites his friends.
To measure customer loyalty there is also a metric called Net Promoter Score. To calculate this metric, you need to conduct a survey among your users. Ask them to estimate the probability if they can share information about your product with their friends on a scale from 0 to 10.
Those who answered 9 or 10 - the so-called promoters - your most loyal friends. They are happy to tell a friend about the product that they like. Those who put the evaluation from 0 to 6 are detractors, they don’t appreciate you, and vice versa, will spread negative information about your product.
By subtracting the percentage of detractors from the percentage of promoters, you get the desired Net Promoter Score, which characterizes the level of loyalty from your users to you.
Two recommendations on using Net Promoter Score:
- Conduct such surveys on a regular basis. For example, every 3 months. Then you will be able to evaluate the dynamics of user loyalty towards your app and to understand whenever you’re moving in the right direction.
- Segment users in every possible way and evaluate Net Promoter Score for each segment separately. That’s how you can understand more whom you are making the game for, who are your fans and opponents. And based on that, you will be able to make better decisions in the future.
Hopefully, metrics we have described will be useful. And if you share information about these metrics with your friends, your Sticky Factor and Net Promoter Score will be close to 100% 😉
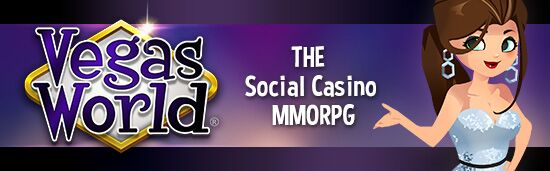
Vasiliy Sabirov, lead analyst for devtodev, has more than 7 years of game analytics experience - started as a payment analyst, now focused on detailed game economics analysis.
Comments
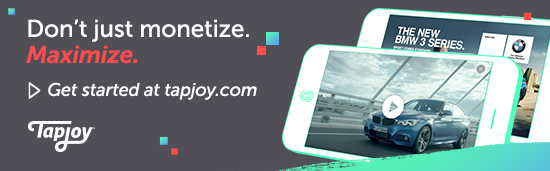